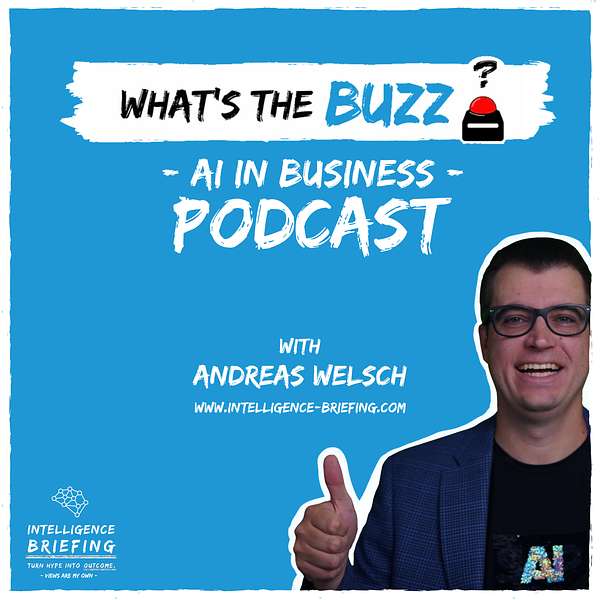
What’s the BUZZ? — AI in Business
“What’s the 𝘽𝙐𝙕𝙕?” is a bi-weekly live format where leaders and hands-on practitioners in the field of artificial intelligence, generative AI, and automation share their insights and experiences on how they have successfully turned hype into outcome.
Each episode features a different guest who shares their journey in implementing AI and automation in business. From overcoming challenges to seeing real results, our guests provide valuable insights and practical advice for those looking to leverage the power of AI, generative AI, and process automation.
Whether you're just starting out or looking to take your efforts to the next level, “What’s the 𝘽𝙐𝙕𝙕?” is the perfect resource for staying up-to-date on the latest trends and best practices in the world of AI and automation.
What’s the BUZZ? — AI in Business
Teach Your Data Scientists About The Business (Guest: Mark Stouse)
In this episode, Mark Stouse (CEO, Proof Analytics) and Andreas Welsch discuss teaching your data scientists about the business. Mark shares his perspective on connecting data science & AI teams with their peers in the business and provides valuable advice for listeners looking to bridge communication gaps, manage expectations, and focus on AI-driven business outcomes.
Key topics:
- Identify the challenges AI and business teams face when working together
- Improve communication between AI and business teams
- Understand the impact when data scientists have more context about the business
- Learn how AI leaders support their team members to upskill
Listen to the full episode to hear how you can:
- Develop an understanding of the business context and connect with business leaders
- Shift from precision to pragmatism
- Deliver business value quickly
- Operationalize data science & AI quickly and adapt to the fast-paced business environment
Watch this episode on YouTube:
https://youtu.be/WE7sopcsFII
Questions or suggestions? Send me a Text Message.
***********
Disclaimer: Views are the participants’ own and do not represent those of any participant’s past, present, or future employers. Participation in this event is independent of any potential business relationship (past, present, or future) between the participants or between their employers.
Level up your AI Leadership game with the AI Leadership Handbook:
https://www.aileadershiphandbook.com
More details:
https://www.intelligence-briefing.com
All episodes:
https://www.intelligence-briefing.com/podcast
Get a weekly thought-provoking post in your inbox:
https://www.intelligence-briefing.com/newsletter
Today, we'll talk about how you can help your data scientists learn about the business that they support. And who better to talk to you about it than somebody who's really passionate about doing that? Mark Stouse. Hey Mark, thanks for hopping on.
Mark Stouse:Hey, thank you so much for having me.
Andreas Welsch:Why don't you tell our audience a little bit about yourself, who you are, and what you do?
Mark Stouse:I'm a business leader long time, large company marketer, CMO, and very large companies like Honeywell Aerospace. For 20 years have been really into leading and championing the use of analytics-led decision making in those functions that I led and in those businesses that I was a part of. Today, I'm the CEO of Proof Analytics.
Andreas Welsch:Thanks for sharing. So definitely sounds like there's some good data science work going on under the hood as well. So really glad to have you on and learn more from you today.
Mark Stouse:Yeah, absolutely.
Andreas Welsch:Mark, should we play a little game to kick things off? What do you say?
Mark Stouse:Sure, absolutely. Why not?
Andreas Welsch:Alright, good. This one is called In Your Own Words. And when I hit the buzzer, the wheels will start spinning. When they stop, you'll see a sentence. And I'd like you to complete that sentence in your own words with the first thing that comes to mind and why. To make it a little more interesting, you only have 60 seconds on the clock. And we're very curious to see what you come up with. Are you ready for What's the Buzz?
Mark Stouse:Yes.
Andreas Welsch:Here we go. If AI were a tool what would it be? 60 seconds on the clock? Go!
Mark Stouse:A magnifying glass.
Andreas Welsch:Why would it be a magnifying glass?
Mark Stouse:It tends to illuminate things that are not obvious. And if you're not careful, it can also set them on fire.
Andreas Welsch:That's a pretty good and concise answer. I really like that. Magnifying glass. That's a new one. I think also AI is a bit under the magnifying glass as well itself, right?
Mark Stouse:Very, much.
Andreas Welsch:If you, follow the news and everything that's going on and I'm sure business leaders themselves are using that magnifying glass to see where are the opportunities. Really love that answer. Now to go more to the ones that we had prepared for our talk. You must be seeing that as well in your business and in your work. And I'm sure you've seen that over the course of your career, where it's been data-driven, where you've worked with folks in analytics functions, in data science, in AI. What do you see are the key challenges when on one hand, these technical data science, AI teams work together, but on the other hand, business. What are the key challenges?
Mark Stouse:We can just start with the fact that there's not a common language, and that a lot of the phrases that are thrown around, data-driven being one of them, actually are really problematic. A lot of these fundamentals get lost in the shuffle after a while. Data is always and only about the past. So saying that you are data-driven is the equivalent to driving your car on the freeway at 100 miles an hour looking only in the rearview mirror. And it's not going to be very long before you have an unpleasant experience doing that, right? Really, we need to be analytics-led. Now, I'll be the first to say that analytics-led, doesn't roll off the tongue. The alliteration is not as cool as data-driven, right? But it is factually far more accurate. And it acknowledges the fact that data is a raw material that, like crude oil, if you pour it straight into your car, it's not going to run. You have to refine it further to gain utility to actually drive something. Make it happen. The same thing is true for most data, particularly in a business context where almost all of the questions that business leaders have are around causality. If you're, not reducing, data, if you're not distilling data by mixing it in with other data to catalyze it and get it into a multivariable state where you're starting to see causality. You're not going to be answering most business leaders questions. Likewise, I think if you try for a super academic, cult of precision approach to data science in a business context, you will be perpetually frustrated, and perpetually frustrating to the business. They couldn't care less about the 95 percent confidence score, for example. They are operating in routinely in decisions that if modeled after the fact, and I have done a lot of that kind of work in the past, routinely come in the high teens to high twenties in terms of confidence scores. And that's their norm. That's their routine situation. So the way that they're looking at the effect of great analytics is that if you can get me into the 30s, man, if you can get me into the 40s, if you can get me to a point where every time I have to remake that decision, I'm a half a point better than I was the day before. If you're a business leader and you have to make the same decision every morning for 365 mornings. And a half a point improvement per day is something that's achievable consistently. By the end of the year, on an annualized basis you're bumping what, almost 2, 000 percent improvement, right? By anybody's standards, that is huge. If you cut it in half, it is still huge. And if you cut it in half again it's pretty damn amazing. I think that that, is the kind of thing that we have to really be aware of. And the last thing is the, issue of latency and scalability and speed and understandability. These have nothing to do with the math and everything to do with the way that analytics operationalize into the business. And if data science teams aren't executing against those priorities, if they are loving complexity too much, if they are eight years in still working on data management, and not able, not ready yet to help the business with causal questions. That's a huge problem and we're seeing actually a lot of that right now, to the point where a lot of you guys may or may not remember this, but 20 some odd years ago, after Y2K, a lot of enterprise IT organizations got pulled under finance, not because finance thought that it knew more about IT than IT. But because they said what happened with Y2K was so ridiculous that we are going to put a new culture, we're going to graft a new culture onto enterprise IT, and it's going to be a T-shaped perspective. It's going to be a business first, let's call it finance first, perspective on technology. And 20 some odd years later, they're doing the same thing in many cases with data science teams.
Andreas Welsch:I think you hit on a number of excellent points. You mentioned something that I think is especially important when you look at data science problems through a technology lens. And that's it's not about getting to that very last percentile of optimization and, our love and desire for big numbers and huge improvements, right? To your point, whether it's 95 percent accurate or double digit X improvement in many cases in realistically and in business, to your point, even a fraction of a percent or a few percent can have a huge impact in absolute numbers. So I think it also depends on the metric and the unit of measure that you take as your basis to see how successful something is. I've seen that before too, right? It's where we're able to automate 80 or 90 or 95 percent of this or that process. If you're at 10 percent or 20, 30 is actually a really good step up.
Mark Stouse:What's also really important to say is that the origin of the 95 percent confidence score is physical sciences. As soon as you get into the social sciences, meaning anything having to do with human behavior at all, the best that you can expect is 50ish. For a lot of really super pure, data scientists, they won't even consider 50 percent confidence scores to be worth anything at all.
Andreas Welsch:Building on that and building on communication, or maybe not speaking the same language. It's a known issue and a known fact, right? Business and data science teams don't always communicate well, and you already alluded to it. They're not speaking the same language. But I wonder, from your vantage point, why hasn't that been solved yet, if it is a known issue?
Mark Stouse:I think it's because there's a huge cultural gap. Can we just talk frankly here for a second? These are two groups of people who are so far apart in the way that they see the world that they would not choose most of the time to have cocktails with each other. This is a little bit of an extension of what we all went through in high school: the jocks versus the nerds and all that kind of stuff, right? And never the twain shall meet. This is an adult version of that problem. And part of it is the language. So one of the things that I love about hanging out with data scientists is that they are among the most argumentative people on the face of the earth. They will pick up the cudgels on how many angels dance on the head of a pin kind of argument at the drop of a hat. They're ready to go. And I happen to personally enjoy that kind of sparring as well. So even though I'm a business guy and I'm ultra pragmatic, I can, totally hang with that. Most business leaders just aren't even remotely interested in that and don't have the time, don't have the inclination, and think that the whole thing is just ridiculous, right? So there's a numerator denominator relationship here. And the business is, the permanent denominator. So the business is not going to change to become more like what data science wants it to be. It's going to have to be the other way around. So that's either something that you're okay with as a data scientist, and you can make those adjustments, or you can't. And I'm not saying that you should be one or the other. I think that you definitely, you owe it to yourself and a lot of other people to know what is true for you and act accordingly. Because yes salaries for data scientists inside of companies can be really good. But what goes with that is the expectation that you're going to do what they want you to do. And that you're going to, you're going to meet the goals and expectations and be malleable to their cultural perspective around business.
Andreas Welsch:Mark, that brings up another important point, I think, and that's the role of the leader. There's certainly so many different requirements and different expectations. And you're being pulled in so many different directions, if you're managing an AI or data science team, right? And on one hand, you are most likely technically savvy or have been in a data science role yourself before. So you have a good understanding of this side of the business. But at the same time, you need to manage expectations up towards your management, which could be a CIO, CDO, CTO, chief Analytics Officer type role, and collaborate across with different business units, with different business leaders. Maybe you need to increase AI literacy and shape that mindset that AI is something important, that it helps you, right? All these kind of things. And then on top of it, you also need to coach your team and give them guidance. Hey, if you're working with this business team in a specific domain, here are things you need to be aware of, right? Or maybe coach them before they go into a presentation. Yes, there are some metrics and some numbers and some more technical results that we all know of. But to your point your business peer is not going to care so much about whether it's 98. 0 or 98. 2 percent accuracy questions. What am I getting out of it? So what other challenges or requirements do you see there for leaders to help their teams move up and have that conversation on a different level beyond just pure technology?
Mark Stouse:So you're absolutely right. And one of the things that I end up doing a lot in different companies is helping with that. There's a lot of CDOs currently in those roles that are not very T shaped themselves, meaning they don't really understand very much about the business themselves. And so it's really hard for them to guide their teams effectively in that respect. So here's some key rules that I have found work all the time, no exceptions. It's great if you have a switched on CDO. It's great if you are that switched on CDO. But these apply at every level, because you're going to have peer to peer conversations about data science and business. It's not all going to be hierarchical. Okay, so number one, if you sit there and wait. to be directed by the business, you've already lost. So the way that you have to do this is you have to sit down with the business leader that you're closest to, that you're working with, and say, hey, what are the top 10, top 20, top 50, whatever the number is, questions or decisions that keep you up. That if we were able to give you greater clarity about what's actually happening and all the variables that come together to produce a particular outcome related to that decision or that question, that it would really help you. He or she's not going to understand how the math and all that kind of stuff, and you don't have the time to try and make them data literate, right? That is a much longer term project. And again if you try to start out there, you will end up spending a year on that and, you will have done nothing else and you will have nothing to show for your work, right? Bad idea to start there. You have to push some of these balls together at the same time to varying degrees, knowing that the most important thing is that you give them something that they find really valuable. And you do it again and again. And that is what It is called earning your right to be heard and then earning your right to gain additional investments, earning your right to do even more, to ask even more, to become even more indispensable to the decision making process of the company. But all that has to be built just like a house, right? You can't just do the beautiful interior and then build the exterior piece. That's not the way it works. So you got to really start very basically. When we are onboarding a new customer, we actually chair a meeting with usually it has a lot of the C suite leaders in this meeting. And we ask them that very same question. What do you most want to know? And then we start working with the internal team to help them build out models of causality in Proof that then become effectively nearly autonomous in terms of recalculation of the model and it becomes an on demand business GPS in the sense of what's going on any given question that's being posed. The reason why I'm talking about that briefly is that it is an automated process that is the process that you guys need to be really thinking about when you're working with the business.
Andreas Welsch:And I can also see that it norms the C-Suite or those participating, right? Are these the questions we really want to ask and have an answer for? Are these the most critical ones and drive that alignment and that the discussion, right? And I'm looking at the chat and I see Sujata says at the end of the day it all comes down to return on investment.
Mark Stouse:Let me amplify on that just a little bit. Return on investment is also historical. By the time you can compute it, you can't do anything about it. That's what makes it historical. So what really matters is being able to forecast ROI. And then compare that all throughout the process to the actuals that are being delivered at any given time. Again, this is very much like a GPS, right? The GPS says this is where you should be on your route. And we've all seen this, particularly if you're walking in Manhattan. The dot doesn't necessarily represent where you actually are. So that has to be adjusted. That has to be brought into a place of fidelity. Occasionally you have to reroute in the face of changing conditions. So the route that you were on could have been exactly the best way to get to your business goal. And then stuff in the world, in the marketplace, your competitors, whatever, changed. And that's not the best route anymore at least not right now. So you gotta go somewhere else.
Andreas Welsch:That makes a lot of sense, yeah. Now look we're coming up towards the end of the show. And I was wondering if you can summarize the three key takeaways for our audience today before we wrap up.
Mark Stouse:I would say that the three key takeaways are, you have to lead the business in your area. They are generalists. You are a specialist in data science. But if you talk to them in data science terms, you're not going to connect. You have to be able to talk about data science in business terms, what it's going to get them from a business benefit. I think you also have to completely readjust away from a cult of precision into something that is more like a cult of pragmatism. I think that is super key. Where in the companies that I'm aware of where data science teams are in really bad trouble with the business, it's because they spent all this time, money, and effort on very complex, arcane data management, which a lot of businesses regard as roads to nowhere. Why? Why did they regard them as roads to nowhere? Because when they turned around 18 months ago and asked the data science teams for help understanding the dynamic events that were changing and what that needed to mean for the way that they conducted business, the data science teams, a lot of times, told them, hey, we're not ready to do that. We're still working on the road to nowhere. You just can't do that. That's like signing your own death warrant professionally speaking, anyway, at that company. And then the third, I would say, is you have to see this not from a mathematical process, although we all understand that is important. But if you can't operationalize analytics at the clock speed of the business, there's something in the military called the OODA loop. It's the speed with which you observe, orient, decide, and act. It's an Air Force term. There's another one called VUCA. Right? Talks about the volatility and uncertainty of the environment, right? If you can't OODA really fast in a VUCA environment, which is the kind of thing we're all in right now, you are screwed. Okay? So if you are modeling, if you're creating models that are only updated once or twice a year, that is not going to cut it in this day and age. It's just not. And those would be the three big points that I would make.
Andreas Welsch:Perfect. Thank you so much, Mark. Like I said, we're coming up to the end of the show. Thank you so much for joining us and for sharing your expertise with us. And for those of you in the audience, thanks for learning with us today. Mark, it was great to have you on.
Mark Stouse:Hey, thank you. Thanks guys, and I appreciate the questions.
Andreas Welsch:Awesome.